Automation technology is extremely vital for wheat food security, enhancing breeding efficiency and food production. Wheat, as one of the most important food crops, provides approximately 20% of the world's protein and carbohydrate intake and bears the burden of global food security. Not only that, wheat also has a variety of uses, such as industrial raw materials, biofuel, and animal feed. However, as the world develops, the growth in wheat production has not kept pace with the growth in demand. For this reason, automation technology has been fully utilized to improve breeding efficiency and to cope with the global food crisis. More specifically, automation technology can use modern computers to replace manual statistical analysis of crop phenotypes (including height, color, number of ears, and other relevant phenotypes), thus reducing labor and time costs and achieving efficient breeding. Selecting varieties with desirable traits through high-quality and automated counting is an essential process in breeding. Wheat yield, as one of the most important excellent traits, is determined by three elements: the number of wheat ears per unit ground area, the number of grains, and the weight of 1,000 grains. Traditional breeding methods use manual counting to complete the number of wheat ears, which suffer from low efficiency, high time cost, and high error. Therefore, automated counting is indispensable for improving breeding efficiency and saving human resources. In pursuit of achieving high-quality and automated counting, researchers have begun to adopt image-processing techniques to recognize wheat ears. With the rapid development of deep learning, position-supervised methods, including box-supervised and point-supervised wheat counting approaches, have received wide attention. On the one hand, bounding boxes are employed to select and quantify the number of wheat ears in terms of box-supervised wheat ear counting methods. On the other hand, predicting the density map of wheat ears can achieve counting in the case of point-supervised wheat ear counting methods. The above methods have achieved excellent results in wheat ear counting, while they require training on high-cost position-level images. To address this problem, we propose a count-supervised multiscale perceptive wheat counting network (CSNet, Count-supervised Network), which aims to achieve accurate counting of the wheat ear via quantity information. Specifically, in the absence of location information, CSNet adopts MLP-Mixer to construct a multiscale perception module with a global receptive field, which implements the learning of small target attention maps between wheat ears features. The superior performance indicates that our approach has a positive impact on improving ear count and reducing labeling costs, demonstrating the great potential for agriculture counting tasks.
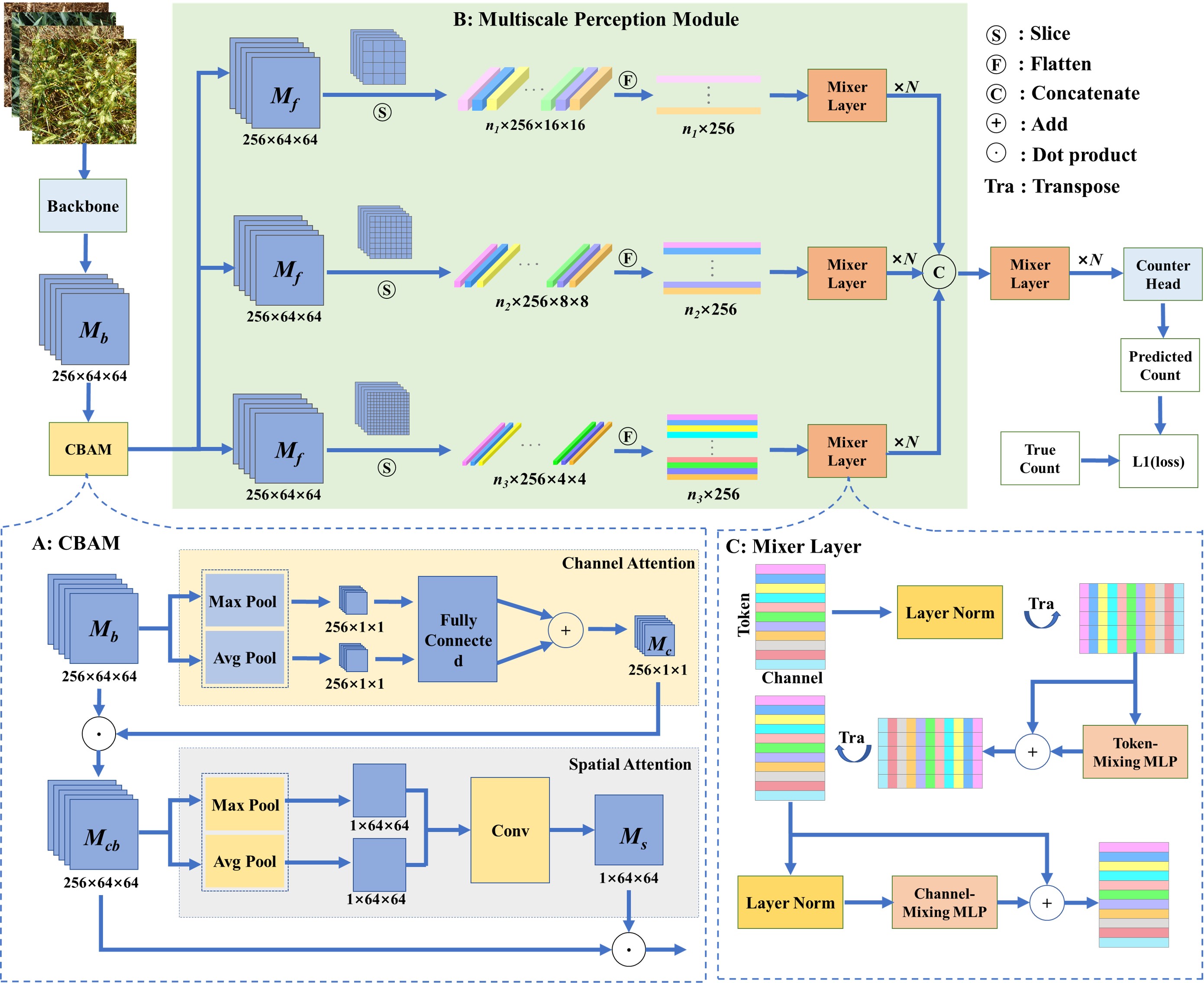
The overall architecture of CSNet